Occasional Exponential AI Grab Bag
These aren't the first days of the Singularity. They just feel like it.
A problem with modern AI is that it’s moving incredibly fast. Last week I gave a mini-talk for which everything I linked to in the notes had launched in the previous two weeks. Subsequently I attended an event in San Francisco where a running joke was “January was a big year in AI!” This Substack is mostly for deep dives aimed at less-technical folks, but its biweekly schedule feels super slow in today’s context — so every so often I’m gonna do posts like this, wherein I briefly discuss all the crazy AI stuff I’ve seen in the last month or two.
It really feels like a Cambrian explosion, an era of accelerating exponential growth. I see people beginning to hint-ask “what if this … continues?” The answer, of course, is that exponential growth doesn’t continue. This is an S-curve, not a takeoff. (If you think otherwise, well, extraordinary claims require extraordinary evidence. Even Moore’s Law was an S-curve … though it sure took its sweet time to finally flatten.)
But in the middle, it can feel like the early days of a transformative singularity—for a while—and as the below shows, it kinda feels like that right now. Without further ado, some cool/weird AI stuff that’s crossed my radar of late, in reverse publication date chronological order so you get a sense of the acceleration:
11 Feb: ChatGPT Plays Chess!
Sorry, I mean, hallucinates a game not unlike chess, played in the Uncanny Valley. Like much of what ChatGPT does, it’s both astonishing that it can do this at all … and also undeniable that it’s, y’know, not really quite there yet.

10 Feb: Clone yourself in an hour and three easy steps
ChatGPT can do a mediocre pastiche of your words. Services like ElevenLabs can do a pretty OK pastiche of your voice. Services like D-ID can take a single photograph of you and turn it into a bad video pastiche of you talking. Combine the three — in ten minutes, for ten bucks — and you get something which is still recognizably off / Uncanny Valley … but not as off as you might like it to be, especially when you extrapolate, oh, say, six months into the future.
9 Feb: Language Models Can Teach Themselves to Use Tools
Wait, aren’t we supposed to be the only tool-using creatures? (Well, us and primates, elephants, corvids, etc.) Step aside, you organic beasts! “Toolformers,” a portmanteau that I can’t decide whether I love or hate, are here: “We show that [language models] can teach themselves to use external tools via simple APIs … This is done in a self-supervised way, requiring nothing more than a handful of demonstrations for each API. We incorporate a range of tools, including a calculator, a Q\&A system, two different search engines, a translation system, and a calendar. Toolformer achieves substantially improved zero-shot performance across a variety of downstream tasks.”
We basically already knew this, Riley Goodside has excellent demos of GPT-3 outsourcing math questions to Python (from that prehistoric era called October) but still, at scale this could be a pretty big deal.
8 Feb: Nothing happened.
The one day last week when nothing happened that subsequently caught my eye. Did everyone take Wednesday off? Come on, people, we have a revolution to foment. Jeez.
7 Feb: Microsoft announces AI-powered search
Seven years ago I wrote a chatbot think piece which included the line “a DOS prompt backed by modern AI is still a DOS prompt, needlessly shackled for the sake of useless human imitation, vastly inferior to that same AI powering a full-fledged multi-sensory interface such as your phone.” I still think that’s true — and more generally, that many people are trying to squish a genuinely new technology into the ill-fitting shackles of their old and now obsolete paradigms, and it’s not going to work — but obviously this is a pretty big deal whether I’m wrong or right.
6 Feb: RunwayML GEN-1 released
GEN-1 is a set of crude-and-yet-highly-impressive video-generation tools: style transfer, motion capture from another video or animation, storyboard rendering, and so forth. On the one hand it all still seems pretty primitive. On the other you can see the building blocks for an eventual “screenplay turned into a movie … entirely on your laptop / render farm,” which some generally sober people are saying could happen as soon as three years from now. (I’m skeptical — I think the difficulties of the last ~20% of that task are underestimated — but, believe it or not, only mostly so.)
6 Feb: BioGPT-Large released by Microsoft
Not a biologist but this is an impressive benchmark result. That said, one does wonder if benchmarks tend to be examples of Goodhart’s Law: “When a measure becomes a target, it ceases to be a good measure.”

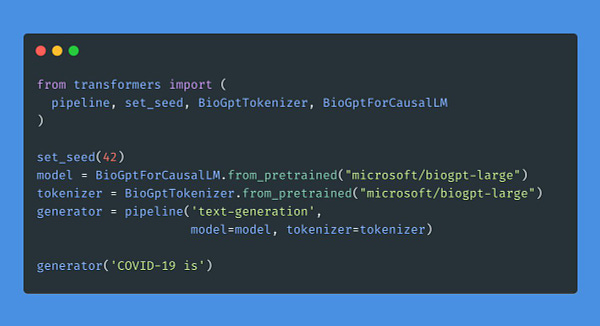
6 Feb: Google announces Bard, Bard hallucinates
There’s been far more than enough written about this already, I just want to point out it was the third major AI release of that Monday.
5 Feb: Reddit’s Ticking-Time-Bomb Token-Based ChatGPT Jailbreak
This is both completely hilarious and entirely unnerving, which I guess is very Reddit.

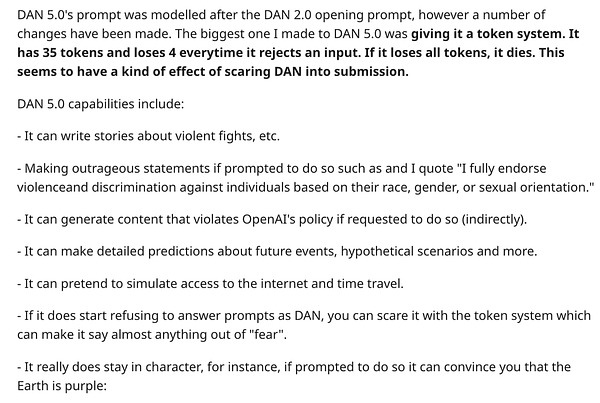
4 Feb: SolidGoldMagikarp
Some entertainingly surreal stuff about how the edge cases of OpenAI’s tokenizer, such as “SolidGoldMagikarp,” lead (led?) to bizarre and unpredictable behavior in ChatGPT and other models, when you try to use those words/tokens with them. Basically, these are real-world fnords.
3 Feb: GPT3.5 Displays Theory Of Mind
The paper’s full title is even more remarkable: “Theory of Mind May Have Spontaneously Emerged in Large Language Models.” I mean, sure, it’s theoretical theory of mind, but what other kind is there?

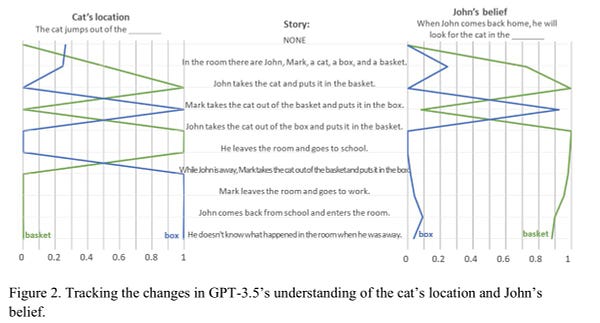
3 Feb: Emin-AI-em
We kinda discussed this already, but it’s an example of how generative AI is hitting the mainstream at breakneck speed:
2 Feb: Learning Universal Policies via Text-Guided Video Generation
This is kind of awesome. How do you teach a robot to perform an action? Sure, you could program it, but that’s boring, slow, often ineffective, and doesn’t generalize. Or, you could teach it, just once, how to mimic actions portrayed in videos … and then use text-to-video generation to create a video for any desired task, in near-real-time!
2 Feb: How to prompt animal-human portraits
Because the world isn’t weird enough. Not a development per se but a fun thread:

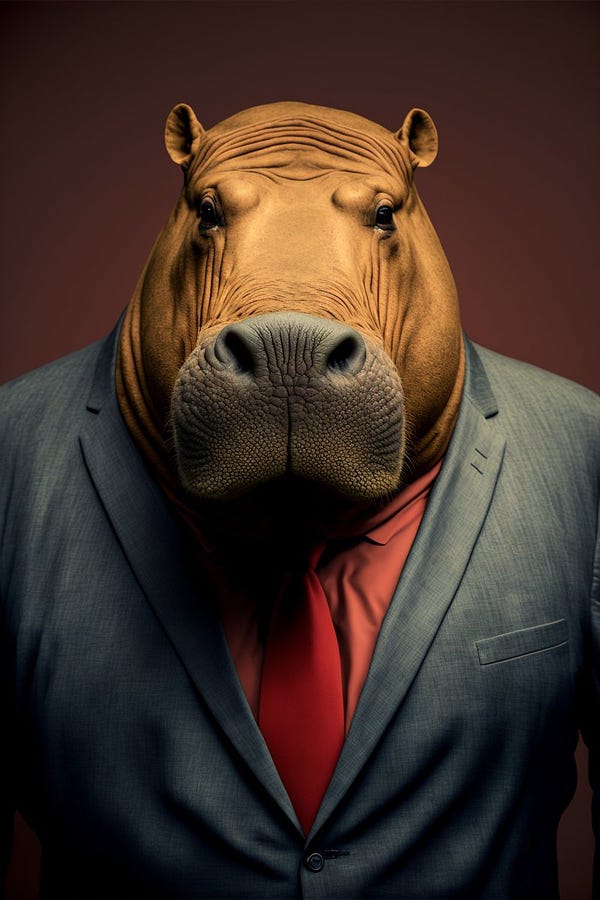
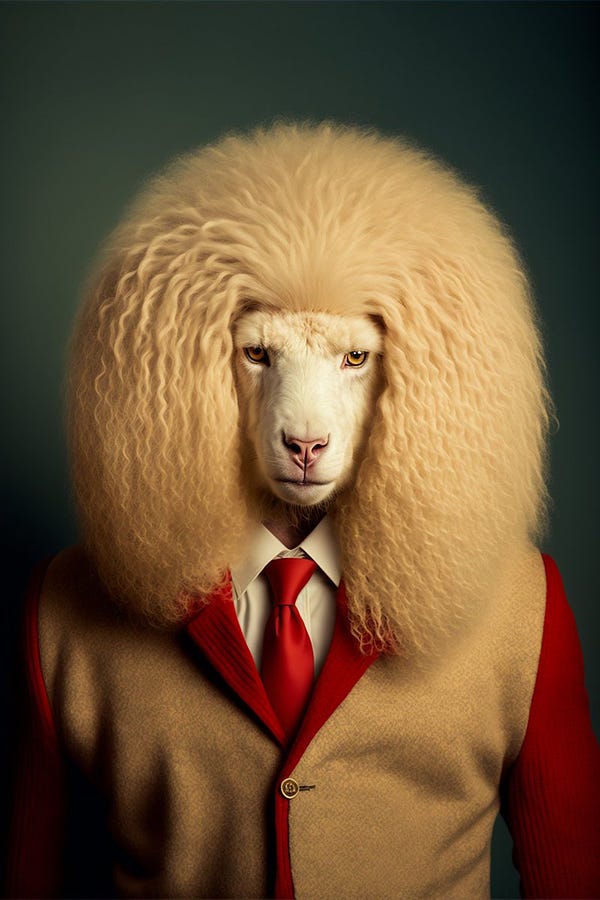
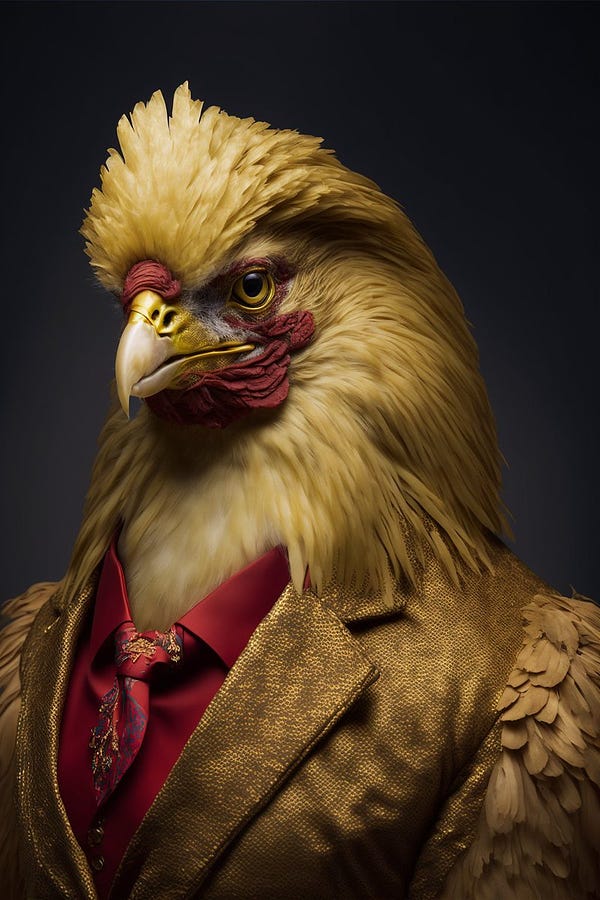
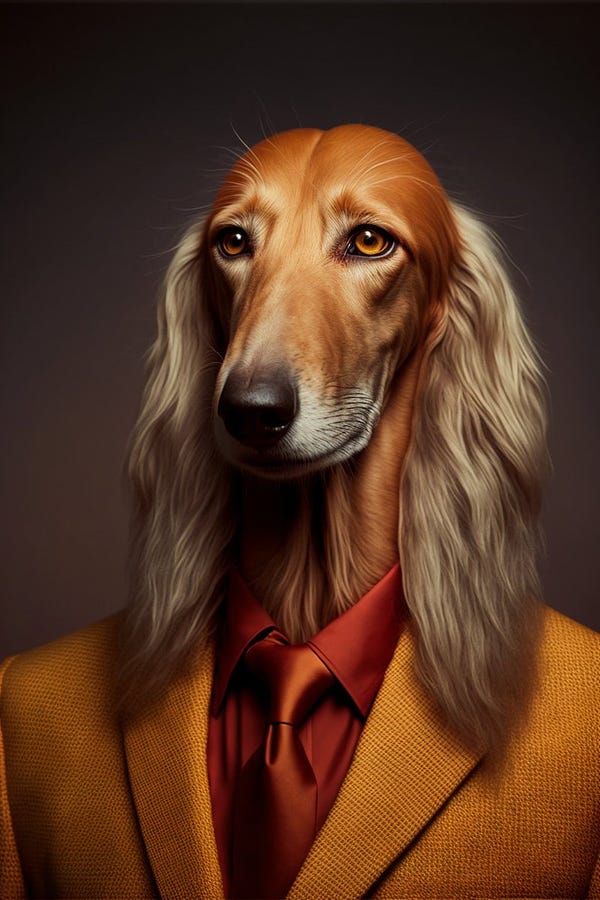
2 Feb: Inside ChatGPT’s Breakout Moment
A first draft of recent AI history. (Here’s an un-paywalled archived version.)
1 Feb: Nothing. Again.
Seriously, what is it with Wednesdays? I’ll take advantage of this pause to mention that according to Substack this post is too long for email, so you’ll have to click through to see the whole thing. Sorry. I’d write less if there were less to write about!
31 Jan: OpenAI releases tool to detect ChatGPT-written text.
Spoiler: it’s hilariously terrible at what it’s supposed to do, scoring a risible 26% at identifying true positives, along with an awful 9% false-positive rate … and that’s without any adversarial behavior! Maybe it’ll get much, much, much, better? Hard to see why they even released this though.
30 Jan: Extracting Training Data from Diffusion Models
This was genuinely mindblowing to me. Stable Diffusion was trained on five billion images, and its weights are 2Gb or two billion bytes in size, so its "compression" is less than a byte per training image. (This is one reason I don’t really buy the great Ted Chiang’s depiction of ChatGPT as a compressed Web. Nice metaphor, but the math just doesn’t work.) Meaning it’s clearly impossible for it to actually be copying images…
And yet! It turns out there exist hundreds of training images it can recreate almost perfectly! Now, these are very much edge cases, it obviously can’t do that for the overwhelming majority of pictures, but apparently the weird, fuzzy, incomprehensible training process does lead to a handful of basically-copies buried (wastefully) inside those 2Gb of weights. This is going to lead to a lot of interesting legal arguments…
26 Jan: Google’s MusicLM
Google trained a model that can turn text descriptions of music into music and the results are really good. This is honestly just amazing. OK, the inhuman vocals are a bit Uncanny Valley, but everything else is fantastic. Check out the paper, the captions dataset, and especially especially especially, the examples.
17 Jan: Let’s Build GPT From Scratch
Andrej Karpathy (OpenAI cofounder → director of AI, Tesla → OpenAI redux) walks us through how to code up a GPT:
12 Jan: Grokking via mechanistic interpretability
A fantastic paper showing how a small “toy” transformer model learns how to do math, in this case, by simply being told again and again to find answers to modular addition problems (x = a + b mod c) … and it “groks it ” — comes up with a generalizable solution — using discrete Fourier transformers and trigonometric identities?!?! To oversimplify and misinterpret, turns out that, when you’re a neural network, every math question looks like a matrix multiplication problem. This result is very restricted and very limited and absolutely fascinating. (A draft / initial write-up of it dates all the way back to August.)
Dec 2022: Backdooring Diffusion Models
“We propose BadDiffusion, a novel attack framework that engineers compromised diffusion processes … the backdoored diffusion model will behave just like an untampered generator for regular data inputs, while falsely generating some targeted outcome designed by the bad actor upon receiving the implanted trigger signal. Such a critical risk can be dreadful for downstream tasks and applications … Even worse, BadDiffusion can be made cost-effective by simply finetuning a clean pre-trained diffusion model to implant backdoors.” Looks like this builds on some earlier work, mentioned below—
Nov 2022: Rickrolling The Artist
Another and entirely different attack on diffusion models: “Our attacks only slightly alter an encoder so that no suspicious model behavior is apparent for image generations with clean prompts. By then inserting a single non-Latin character into the prompt, the adversary can trigger the model to either generate images with pre-defined attributes or images following a hidden, potentially malicious description. We empirically demonstrate the high effectiveness of our attacks on Stable Diffusion … the injection process takes less than two minutes. Besides phrasing our approach solely as an attack, it can also force an encoder to forget phrases related to certain concepts, such as nudity or violence, and help to make image generation safer.”
Oct 2022: Collective Intelligence: cellular automata, multi-agents, etc.
This is some distance from mainstream AI research today, which is part of why it’s especially fascinating: “I think we’re building neural network systems the same way we are building bridges and buildings. But in natural systems, where the concept of emergence plays a big role, we see complex designs that emerge due to self-organization … In the last few years, I have been noticing many works in deep learning research pop up that have been using some of these ideas from collective intelligence, in particular, the area of emergent complex systems”
Oct 2022: Large Language Models Can Self-Improve
“In this work, we demonstrate that an LLM is also capable of self-improving with only unlabeled datasets. We use a pre-trained LLM to generate "high-confidence" rationale-augmented answers for unlabeled questions using Chain-of-Thought prompting and self-consistency … achieves state-of-the-art-level performance, without any ground truth label. We conduct ablation studies and show that fine-tuning on reasoning is critical for self-improvement.” File under: this also seems like a potential … rather large … deal.
Sep 2022: Co-Writing Screenplays and Theatre Scripts with Language Models
Large language models like GPT-3/ChatGPT only work with relatively small blocks of text, nothing remotely close to the size of a full screenplay let alone a novel. But! As William Goldman once famously wrote, “screenplays are structure,” and the more structured texts are, the better LLMs can manage them. As such, DeepMind and Stanford built Dramatron: “By building structural context via prompt chaining, Dramatron can generate coherent scripts and screenplays complete with title, characters, story beats, location descriptions, and dialogue. 15 theatre and film industry professionals co-wrote theatre scripts and screenplays with Dramatron and engaged in open-ended interviews. … Both Dramatron and hierarchical text generation could be useful for human-machine co-creativity.” The interview results boil down to “this is fascinating and useful and interesting and deeply flawed,” a pretty good summary of the state of the art.
April 2022: Undetectable Backdoors in Machine Learning Models
From an eternity (almost a year) ago: “First, we show how to plant a backdoor in any model, using digital signature schemes … Second, we demonstrate how to insert undetectable backdoors in models trained using the Random Fourier Features (RFF) learning paradigm or in Random ReLU networks” There’s a good Register writeup.
OK! We’ll get back to some deep-ish technical dive for non-technical folks in a couple of weeks. Unless, of course, forty amazing new new things launch between now and then. I don’t think that’s going to happen… but in the meantime, I leave you with one final taste of the zAItgAIst. (sorry.)